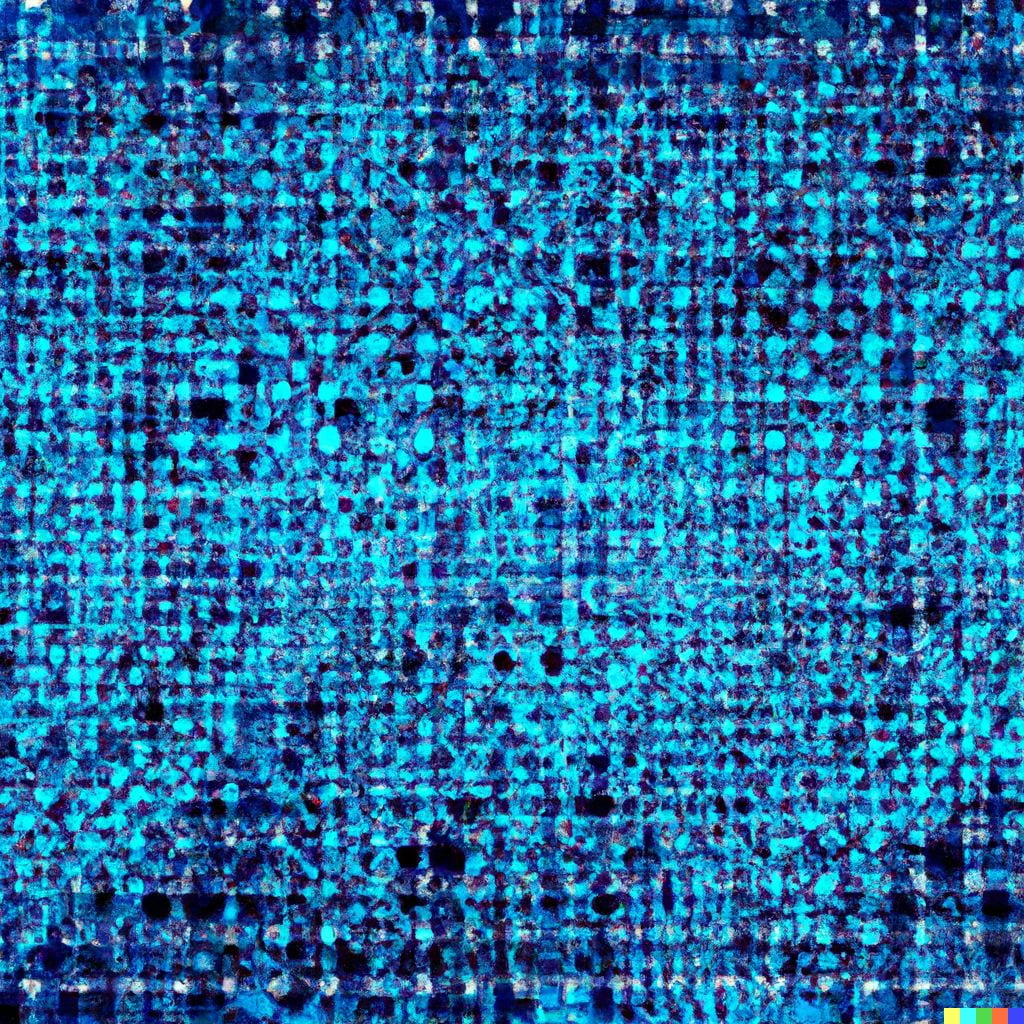
Geometric Machine Learning
We study geometric structure in data and models and how to leverage such information
for the design of efficient machine learning algorithms with provable guarantees.
Our Research
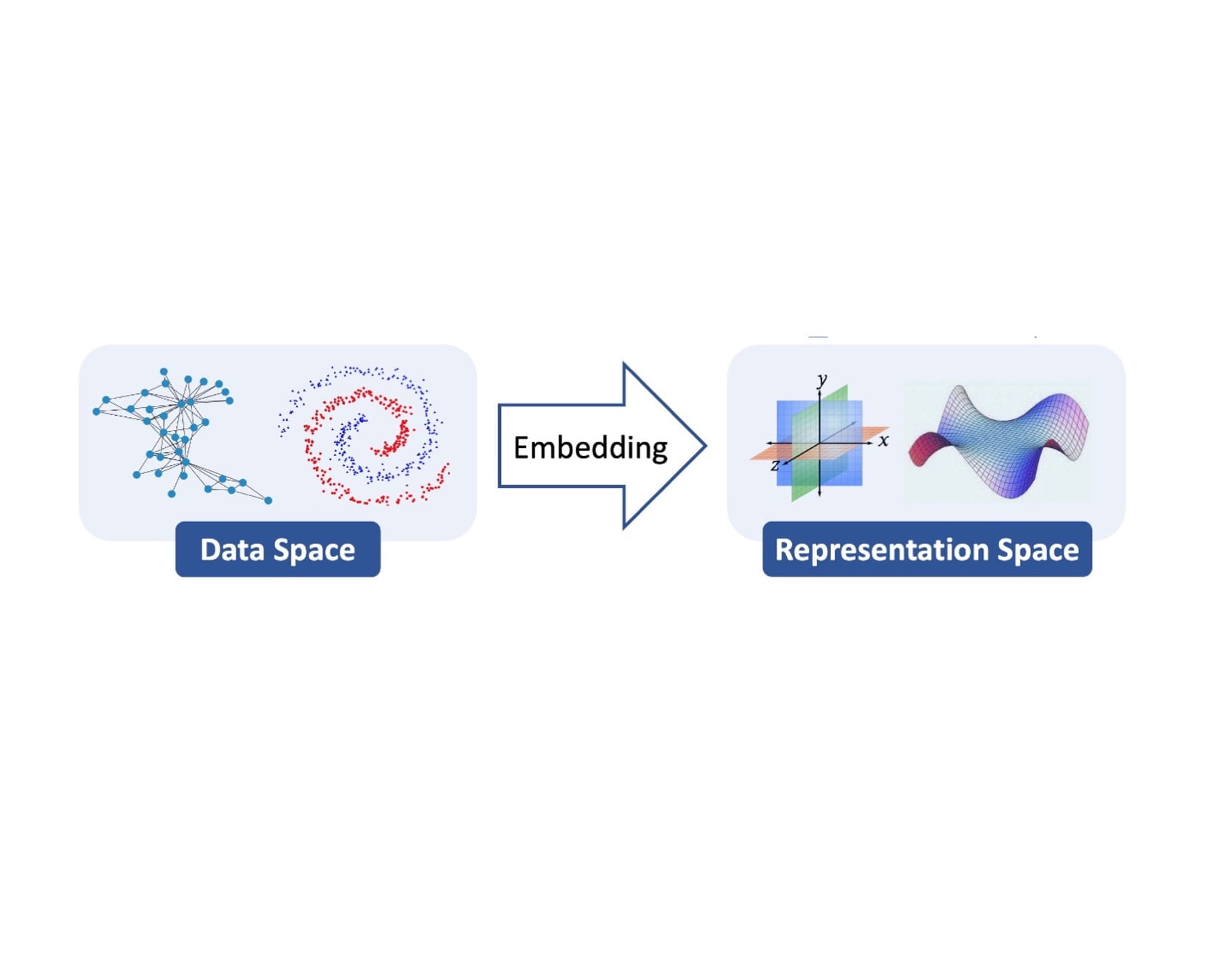
Geometric Representation Learning
Characterizing data geometry and learning data representations in suitable non-Euclidean spaces.

Graph Machine Learning
Leveraging geometric structure for efficient learning on graphs.

Learning Under Symmetry
Designing geometric architectures. Understanding learning-theoretic trade-offs in geometric settings.
Latest News
- [10/2024] Two Spotlights at NeurIPS 2024 and OPT 2024 paperTwo of our papers got accepted at NeurIPS as spotlights. Congrats to Bobak, Jason, and Lukas! B Kiani, J Wang, M Weber: Hardness of…
- [06/2024] Lukas receives Kempner FellowshipLukas was selected for a Kempner Institute Graduate Fellowship. Congratulations!…
- [05/2024] Dean’s Competitive Fund for Promising ScholarshipWe received a grant from the Dean’s Competitive Fund for Promising Scholarship, which will support our research on Riemannian Optimization. Thank…
- [04/2024] Andrew receives NSERC FellowshipAndrew was selected for an NSERC Postgraduate Fellowship. Congratulations!…
- [02/2024] Melanie selected as Sloan FellowMelanie was selected as a 2024 Alfred P. Sloan Fellow in Mathematics. See the Press Release here….